Exploring AI Strategies for Time-Series Forecasting: Benchmarking & Comparative Analysis
Machine learning and AI applications have become increasingly effective for business forecasting and planning functions. But to leverage these technologies for navigating uncertainty, identifying trends, and orchestrating strategies with precision, it’s necessary to understand and leverage the power of time-series data and time-series forecasting applications.
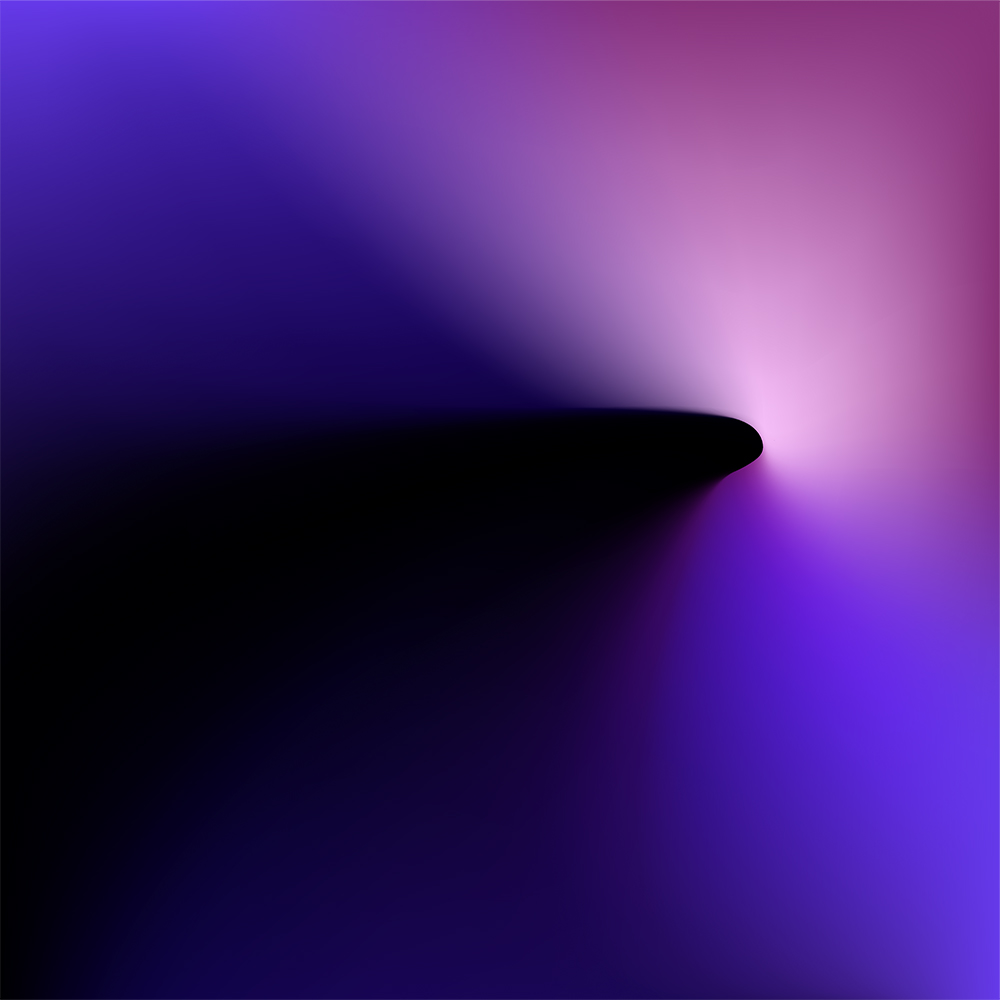
Machine learning and AI applications have become increasingly effective for business forecasting and planning functions. But to leverage these technologies for navigating uncertainty, identifying trends, and orchestrating strategies with precision, it’s necessary to understand and leverage the power of time-series data and time-series forecasting applications.
Time-series forecasting has applications across the entire organization; be it product and sales working together to predict demand and synchronize supply for S&OP planning, finance forecasting cash flow dynamics, or talent teams orchestrating skill sets for optimal workforce planning. No matter the application, the success of aligning often opposing variables – such as supply and demand, or cash and spend – depends on effective integration and analysis of time-series data, such as sales data, financial data, inventory levels, customer engagement metrics, and more.
Historically, businesses have relied on deterministic models for time-series forecasting, piecing together disparate data points with human rules to make educated calculations about future outcomes. But today’s business complexity demands that teams operate in all shades of possibility or probability, a requirement that results in deterministic models, and other traditional approaches, falling flat.
AI-Based Approaches to Time-Series Forecasting: A Performance Comparison
While AI has emerged as a transformative ally for time-series forecasting, AI-based approaches for leveraging time series data are varied. Without a proper understanding of each approach, it can be challenging for business leaders and data science teams to know which of the new modalities is going to be the most impactful for their business.
AI-driven approaches to time-series forecasting that have received some attention include:
- Foundational models
- Internally built / homegrown models
- Ikigai’s time-series forecasting solution, aiCastTM
In a recent technical whitepaper, Time Series Forecasting: Why, What, and How, Ikigai co-founder Devavrat Shah compares these three approaches for performance, accuracy, functionality, production-readiness, cost, and data integrity.
Introducing the Models
Foundational Models
Foundational models, such as Large Language Models ((e.g. GPT-4, Gemini, LLaMA, and Falcon) or emerging time-series specific models (e.g., Google’s recently released TimesFM) attempt to provide pre-built forecasting APIs out of the box. This approach requires using a hosted instance (e.g., GPT from Open AI) or installing and maintaining an open-source model (e.g., Falcon or LLaMa.) To be effective, context data must be provided, and the forecasting mechanism should involve an API call to a foundational model.
Internally Built Models
As one might expect, internally built models allow organizations to create something that is perfectly tailored to their specific use cases and requirements. Usually this is done by fine-tuning an existing foundational model or training an open-source model for time series data (e.g. Prophet from Meta) on specific enterprise data. While some data science teams may relish the notion of more customization and control, the reality is that homegrown forecasting solutions require an astronomical amount of effort from internal teams, especially when it comes to preparing the data, ensuring accuracy, and keeping the model up to date.
Ikigai’s Time-series forecasting with aiCastTM
Ikigai’s comprehensive solution for time-series forecasting leverages generative AI technology to provide robust forecasting for tabular, time-stamped data, along with the flexibility to select the best performing models from a diverse collection. Powered by large graphical models (LGMs) Ikigai’s approach offers domain-agnostic forecasting capabilities that are applicable to any use case or benchmark dataset.
Benchmarking the Models
In the realm of time-series forecasting, a robust toolkit must encompass a range of critical capabilities to navigate the intricacies of data preparation and analysis. Therefore, understanding how to benchmark the performance of AI-driven time-series forecasting models requires a brief education in how models are evaluated. This includes an understanding of key terminology and concepts, such as change points, anomaly, and imputation, and a way to evaluate the effort required for implementation. These subjects are described at length in Shah’s technical whitepaper.
Finally, the key benchmark – the value that most models hang their hat on – is accuracy. To better understand this for the current models, Ikigai conducted head-to-head comparisons between Ikigai’s aiCastTM, a set of open-source models, and Google’s TimesFM. The team used benchmark data – such as the occupancy rate of traffic lanes in San Francisco, the daily exchange rates of eight foreign currencies between 1990 and 2016, and 15-minute electricity loads for 370 households – and looked at the accuracy of the prediction delivered by the model compared to the real outcome, which was reported in terms of Mean Absolute Error (MAE).
In the head-to-head, the results were clear:
- Hosted foundational models had inferior performance compared to other approaches.
- Ikigai’s approach had higher accuracy than Google’s TimesFM foundational model for 12 out of 14 benchmark datasets.
- When Ikigai’s aiCastTM outperformed Google’s TimesFM, it did so on average by 30%.
*For full results and details around the head-to-head tests, please download Time Series Forecasting: Why, What, and How
Beyond Performance: Assessing Implementation Ease and Organizational Readiness
In addition to the performance benchmarks, Ikigai’s aiCast was found to deliver the highest levels of production-readiness; meaning, the solution is fit to be deployed for fast ramp-up and use.
aiCast can be embedded into familiar frameworks and workflows, leveraging API-based integrations to enhance efficiency and effectiveness without disrupting established processes. With its proprietary Expert-in-the-Loop (XitL) technology, internal experts can easily contextualize AI-generated predictions and correct exceptions. With high explainability and interpretability, Ikigai makes it easy for internal teams to understand AI outputs and their implications to avoid misinterpretations and quickly build trust.
In Conclusion
Time-series forecasting remains a critical enterprise endeavor, and enterprise organizations must consider AI-driven alternatives to traditional methods to ensure maximum performance in an inherently dynamic business environment. While foundational models are accessible, and building internal solutions is possible, effective time-series forecasting requires more functionality than most foundational models or open-source models provide. Ikigai’s approach maximized performance & accuracy, while delivering more functionality via production-level ready applications.
If you are interested in seeing the full results of the head-to-head comparison, please request a copy of our technical whitepaper, Time Series Forecasting: Why, What, and How.