Not all GenAI is created equal
When it comes to AI, different use cases require different AI approaches, and each must be evaluated thoroughly to find the right approach that's fit for purpose.
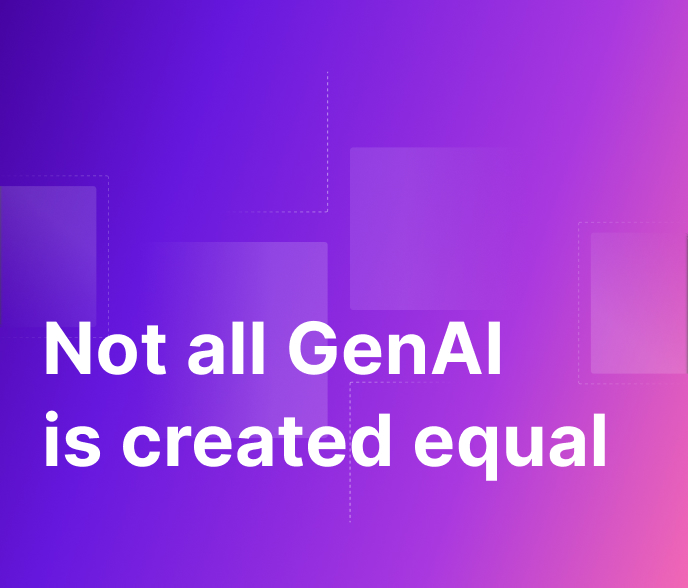
The generative AI hype
The recent hype around generative AI has led to several misconceptions by enterprise leaders as to where the technology fits within their business use cases and what problems it can solve. For many, generative AI has incorrectly become synonymous with Large Language Models (LLMs), resulting in high investment in GenAI projects that are doomed to fail simply because the technology isn’t fit for purpose. The bottom line is that different use cases require different AI approaches, and each must be evaluated thoroughly before investing time, money, and resources.
According to the 2024 Gartner® report, “Using generative AI (GenAI) for the wrong business use cases leads to high failure rates and diminishes the value of AI in organizations. To avoid this, IT leaders can use this guidance to evaluate if GenAI is the right fit for their use case or whether to consider alternative AI techniques.”1
Evaluating business scenarios on a case-by-case basis
While recent generative AI models like GPT-4 and Gemini have made tremendous gains in text-based applications like content generation, chatbots, and knowledge bases, they are not effective in scenarios that require deep numerical predictive and statistical modeling to predict how a given variable will change over time based on one or more input variables (aka regression tasks). As stated by Gartner, “Systematically categorize each use case and evaluate its relative GenAI feasibility. Use cases in the categories of prediction and forecasting, planning and optimization, decision intelligence, and autonomous systems are not currently a good fit for the use of GenAI models in isolation.”2
For these fundamental business planning use cases, organizations primarily depend on enterprise-specific, tabular and time series data that span key areas of the business, including people, products, sales, and budgets. Where Large Language Models (LLMs) lack the ability to effectively operate on this numerical data, new generative AI approaches have emerged, such as Ikigai’s Large Graphical Model (LGM), which is purpose-built to address the unique characteristics and requirements of enterprise data for the purposes of probabilistic forecasting and planning.
Forecasting and planning are the foundation of every business across every industry, impacting use cases such as demand planning, inventory optimization, promotions, risk mitigation, labor scheduling, pricing optimization, transportation, and more. Generating highly accurate forecasts requires sophisticated probabilistic techniques that can respond quickly to change, like supply chain disruptions, macroeconomic fluctuations, weather, and social influences. And to ensure the model outputs are well-understood and trusted by decision makers, explainability must be a core capability of the model, eliminating fear of false outputs, hallucinations, and compliance concerns. As opposed to LLMs, Large Graphical Models are purpose-built to address all these requirements, closing the generative AI gaps in numerical predictive and statistical modeling.
LGMs uniquely address specific challenges related to forecasting, planning, and optimization
LGM-based models can be trained on enterprise data only
As compared to LLMs which require internet scale data to train their models, LGMs can operate on limited, sparse enterprise-specific data. This makes them highly effective for forecasting and scenario planning which depend on historical data, such as sales data, marketing promotions, or inventory, which are by nature in tabular and time series formats.
Inconsistent, inaccurate, and noisy data
Enterprise data is inherently incomplete and messy. Disparate data sources with different nomenclatures, unmatched columns, and missing data are the bane of every analyst, resulting in an average of 80% of time reconciling data instead of generating forecasts and plans. LGMs address this problem by automatically finding relationships across datasets and filling in the missing data via imputation. This allows more accurate forecasts and plans to be generated using less data, less compute, lower training and operating cost – and with greater speed and accuracy.
Ability to adapt to uncertainty
As covered in The Case for Probabilistic vs. Deterministic Forecasting and Planning, AI models need to adapt to today’s uncertain business climate. Rules-based systems - while based on human experience - are rigid and unable to keep up with dynamic changes in customer preferences, supply chain disruptions, and economic variability. LGMs, on the other hand, are probabilistic in nature, creating a range of potential outcomes with associated probabilities, enabling a more data-driven, agile approach to decision making.
Putting the right approach into play
At the end of the day, it’s all about finding the right approach – or set of approaches - for each business use case. It’s important to remember that, despite the hype, Generative AI is only one part of the overall AI landscape, and Large Language Models are only one part of the Generative AI landscape. While LLMs are deservedly having their moment in text and image-based applications, organizations are quickly finding that they are not the solution to their complex and dynamic forecasting and scenario planning use cases that rely on highly accurate numerical analysis of enterprise-specific tabular and time series data. And for that, Large Graphical Models are uniquely fit for purpose.
Learn more about Ikigai and Large Graphical Models here.
1, 2 Gartner, When Not to Use Generative AI, By Leinar Ramos, Ben Yan, Haritha Khandabattu, Gabriele Rigon, 19 March 2024
GARTNER is a registered trademark and service mark of Gartner, Inc. and/or its affiliates in the U.S. and internationally and is used herein with permission. All rights reserved.